Building Better Climate Models
Newly launched center leverages AI for better climate prediction
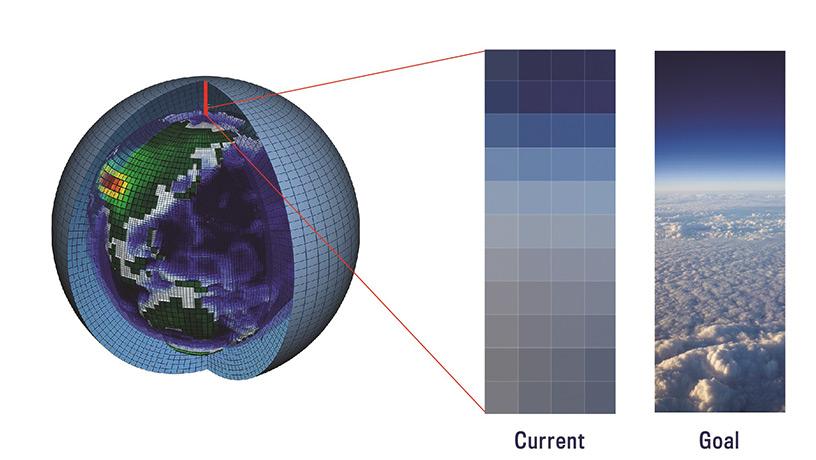
Schematic depicting enhanced clarity in modeling achievable through LEAP techniques.
Our climate is changing. With each passing season, increasingly extreme weather conditions put critical infrastructure, global food supplies, and overall human safety at greater risk.
With billions of dollars and countless lives at stake, how can we more safely navigate this coming age of uncertainty? Enter Columbia’s new center for climate data science—Learning the Earth with Artificial Intelligence and Physics (LEAP)—a $25M initiative to marry machine learning with climate models designed to produce the kind of specific, near-term global climate change projections that have previously been impossible.
It’s an immensely complicated task that requires not just collecting an unprecedented amount of data, but also new tools to make sense of it all, explained the expert panel at “Confronting the New Normal: How Can AI Help Us Prepare for a Changing Climate,” a lively discussion exploring how LEAP combines artificial intelligence, data science, and geoscience to bring the future into sharper focus. Hosted by Columbia Engineering and the Columbia Climate School, the event featured Professor Pierre Gentine, LEAP’s director, and Professor Galen McKinley, the center’s deputy director in conversation with Professor Adam Sobel, director of Columbia’s Initiative on Extreme Weather.
The key will be to home in on the next 10 to 50 years, panelists said. While current climate models show us that global warming is accelerating, they cannot always provide sufficient granular details about potential regional climate shifts—such as sea level rise or droughts—needed to thoroughly understand forces like those that drove Hurricane Ida’s unexpected intensity. Without that detailed understanding, communities and individuals are stymied in their efforts to adapt to climate change, McKinley noted. She describes the limitations of older climate models—including their inability to geographically pinpoint their predictions and that they leave out a lot of new data being garnered from remote sensing and in situ monitoring.
“Machine learning has great potential to harness that data and to help us better understand what’s really going on around the Earth,” said McKinley.
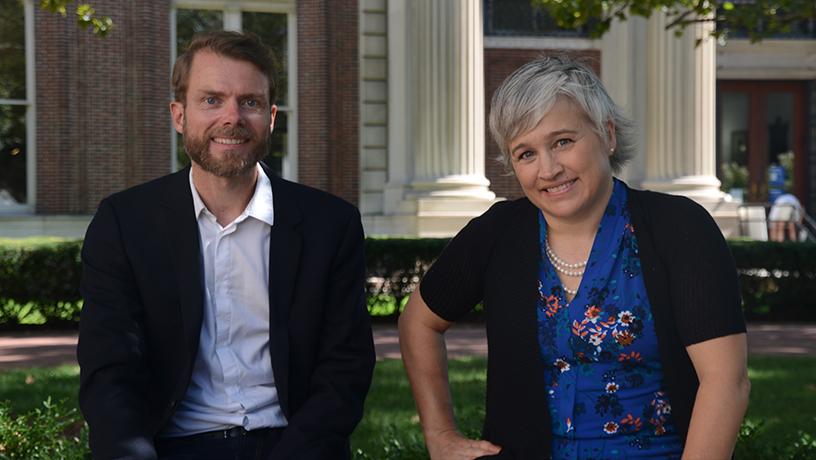
Pierre Gentine, Maurice Ewing and J. Lamar Worzel Professor of Earth and Environmental Engineering, with Deputy Director Galen McKinley, a professor of Earth and Environmental Sciences in the Faculty of Arts and Sciences
LEAP’s new models represent several extreme climate events better, because their method for incorporating machine learning can build in a more nuanced understanding of physical processes, beyond what was possible with older mathematical equations, explained Gentine.
That’s because, due to the complexity of climate processes, models previously had to reduce mathematical equations to their most “idealistic” representations of Earth’s climate systems, ignoring many variables, such as clouds and turbulence. These equations can be further limited in that they may only work under particular conditions. Gentine noted that in some cases machine learning enables the opposite, using massive amounts of data to reverse engineer the physics behind it. He described the process as “throw[ing] data at the algorithm . . . and the algorithm learns by itself without any [pre-programmed knowledge of] physics.” As such, LEAP strives to bridge these two worlds, combining physics and empiricism with data-driven approaches.
That breaks ground in the new field of climate data science, and the center aims to train a whole new cohort of PhD students and postdocs, as well as to inspire the next generation of engineers, both directly and through outreach via Columbia Teacher’s College and the New York City Department of Education.
McKinley and Gentine described some of the initial advantages of their methods. Take those clouds and ocean turbulence. Researchers have already identified how to parse out ocean turbulence on a smaller scale, but until now that information was too data-rich to be accurately modeled simply due to computational limitations. LEAP already has a machine learning approach for running those simulations at high resolution in order to tease out relationships between the large-scale environment and the aforementioned small-scale processes. For other unknowns, such as ice flow and terrestrial ecosystems, LEAP will use “equation discovery,” where researchers feed the algorithm data so as to extract an equation that describes the underlying physics. Machine learning can also help bolster sparse data sets by carefully transposing analogous data across regions. Hopes are that all this adds up to concrete, actionable intelligence in the short term, like predicting the future of crops in different global regions and the various consequences of—and potential solutions for—drought.
“We are already facing that dilemma where we need to predict something we’ve never seen before,” Gentine said. “But we can leverage some of the information we have at those fast time scales.”