New Method Predicts Extreme Events More Accurately
Columbia Engineers develop machine-learning algorithm that will help researchers to better understand and mitigate the impact of extreme weather events, which are becoming more frequent in our warming climate.
MEDIA CONTACT
Holly Evarts, Director of Strategic Communications and Media Relations 347-453-7408 (c) | 212-854-3206 (o) | holly.evarts@columbia.edu
ABOUT THE STUDY
JOURNAL: PNAS
TITLE: "Implicit learning of convective organization explains precipitation stochasticity"
AUTHORS: Sara Shamekh, Kara Lamb, Yu Huang, Pierre Gentine
Department of Earth of Environmental Engineering, Columbia University, New York, NY, USA
FUNDING: The study was supported by: SS and PG acknowledge funding from European Research Council grant USMILE, from Schmidt Future project M2LiNES and from the National Science Foundation Science and Technology Center (STC) Learning the Earth with Artificial intelligence and Physics (LEAP), Award 2019625 - STC. KDL acknowledges support from LEAP and DOE Grant DE-SC0022323 ``Discovering Physically Meaningful Structures from Climate Extreme Data."
The authors declare no financial or other conflicts of interest.
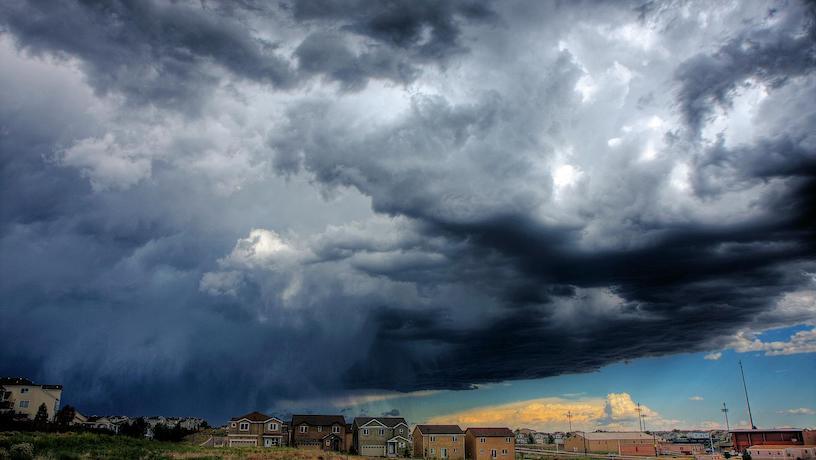
Credit: "Rain Storm Colorado Springs Colorado" by Brokentaco/Flickr is licensed under CC BY 2.0
New York, NY—May 23, 2023—With the rise of extreme weather events, which are becoming more frequent in our warming climate, accurate predictions are becoming more critical for all of us, from farmers to city-dwellers to businesses around the world. To date, climate models have failed to accurately predict precipitation intensity, particularly extremes. While in nature, precipitation can be very varied, with many extremes of precipitation, climate models predict a smaller variance in precipitation with a bias toward light rain.
Missing piece in current algorithms: cloud organization
Researchers have been working to develop algorithms that will improve prediction accuracy but, as Columbia Engineering climate scientists report, there has been a missing piece of information in traditional climate model parameterizations--a way to describe cloud structure and organization that is so fine-scale it is not captured on the computational grid being used. These organization measurements affect predictions of both precipitation intensity and its stochasticity, the variability of random fluctuations in precipitation intensity. Up to now, there has not been an effective, accurate way to measure cloud structure and quantify its impact.
For many years, the scientific community has debated whether to include cloud organization in climate models. Our work provides an answer to the debate and a novel solution for including organization, showing that including this information can significantly improve our prediction of precipitation intensity and variability.
A new study from a team led by Pierre Gentine, director of the Learning the Earth with Artificial Intelligence and Physics (LEAP) Center, used global storm-resolving simulations and machine learning to create an algorithm that can deal separately with two different scales of cloud organization: those resolved by a climate model, and those that cannot be resolved as they are too small. This new approach addresses the missing piece of information in traditional climate model parameterizations and provides a way to predict precipitation intensity and variability more precisely.
“Our findings are especially exciting because, for many years, the scientific community has debated whether to include cloud organization in climate models,” said Gentine, Maurice Ewing and J. Lamar Worzel Professor of Geophysics in the Departments of Earth and Environmental Engineering and Earth Environmental Sciences and a member of the Data Science Institute. “Our work provides an answer to the debate and a novel solution for including organization, showing that including this information can significantly improve our prediction of precipitation intensity and variability.”
Using AI to design neural network algorithm
Sarah Shamekh, a PhD student working with Gentine, developed a neural network algorithm that learns the relevant information about the role of fine-scale cloud organization (unresolved scales) on precipitation. Because Shamekh did not define a metric or formula in advance, the model learns implicitly--on its own--how to measure the clustering of clouds, a metric of organization, and then uses this metric to improve the prediction of precipitation. Shamekh trained the algorithm on a high-resolution moisture field, encoding the degree of small-scale organization.
“We discovered that our organization metric explains precipitation variability almost entirely and could replace a stochastic parameterization in climate models,” said Shamekh, lead author of the study, published May 8, 2023, by PNAS. “Including this information significantly improved precipitation prediction at the scale relevant to climate models, accurately predicting precipitation extremes and spatial variability.”
Machine-learning algorithm will improve future projections
The researchers are now using their machine-learning approach, which implicitly learns the sub-grid cloud organization metric, in climate models. This should significantly improve the prediction of precipitation intensity and variability, including extreme precipitation events, and enable scientists to better project future changes in the water cycle and extreme weather patterns in a warming climate.
Future work
This research also opens up new avenues for investigation, such as exploring the possibility of precipitation creating memory, where the atmosphere retains information about recent weather conditions, which in turn influences atmospheric conditions later on, in the climate system. This new approach could have wide-ranging applications beyond just precipitation modeling, including better modeling of the ice sheet and ocean surface.